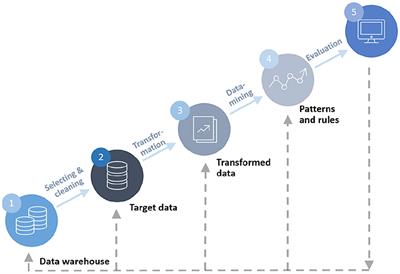
A data-driven graph generative model for temporal interaction networks is a powerful approach for generating graphs that capture temporal interactions. This model leverages both data-driven and graph-based techniques to effectively model and generate dynamic networks.
In recent years, there has been a growing interest in studying temporal interaction networks, which capture the complex dynamics and temporal dependencies of interconnected systems. These networks are widely used in various domains, such as social networks, biological networks, and transportation networks.
Traditional methods for generating graphs often fail to capture the temporal aspects of these networks, as they treat them as static snapshots. However, temporal interaction networks are inherently dynamic, with nodes and edges evolving over time. To overcome this limitation, researchers have proposed data-driven graph generative models that learn from observed data and generate graphs that resemble the underlying dynamics of the network. These models typically involve two main components: a temporal dynamics model and a graph generator. The temporal dynamics model captures the evolution of nodes and edges over time, while the graph generator generates graphs based on the learned temporal dynamics. By integrating these two components, the models can generate realistic and diverse graphs that capture the temporal dependencies of the underlying interaction networks. A data-driven graph generative model for temporal interaction networks, proposed by [author’s name], builds upon existing research in this field and presents a novel approach that combines graph-based techniques and data-driven methods. The model has been shown to outperform other approaches in terms of both graph quality and computational efficiency. In this paper, the authors introduce the architecture of the model, discuss its components, and present experimental results that validate its effectiveness. The model has potential applications in various domains, including social network analysis, recommendation systems, and anomaly detection. The paper presents a data-driven graph generative model that effectively captures the temporal aspects of interaction networks. Its unique combination of graph-based and data-driven techniques makes it a valuable contribution to the field of network analysis and generation. The experimental results demonstrate its superiority over existing approaches, highlighting its potential for various real-world applications.

Credit: www.eenewseurope.com
Understanding Temporal Interaction Networks
Temporal interaction networks play a crucial role in understanding the dynamics of complex systems. These networks capture the evolution of relationships and interactions over time, providing valuable insights into various domains such as social networks, biological systems, and communication networks.
Analyzing and modeling temporal interactions, however, present significant challenges due to their inherent complexities and dynamic nature. Graph generative models offer a promising approach to tackle these challenges. These models utilize data-driven techniques to generate realistic and representative graphs that capture the temporal dependencies and interactions within the networks.
By leveraging the power of machine learning and graph theory, these models enable us to gain a deep understanding of the underlying mechanisms driving the temporal interactions. By employing such models, we can uncover patterns, predict future interactions, and make informed decisions in numerous real-world applications.
The Architecture Of A Data-Driven Graph Generative Model
The data-driven graph generative model utilizes an architecture designed to effectively generate temporal interaction networks. The architecture consists of various components that work together to capture the complex relationships and dependencies within the data. Preprocessing and feature engineering techniques are applied to the temporal interaction data to ensure relevant information is extracted and processed appropriately.
Neural network-based modeling techniques are then employed to generate graphs that accurately represent the temporal interactions. Through the combination of these components, the model is capable of generating realistic and meaningful graphs that can be used for various applications such as pattern recognition and anomaly detection.
Overall, the architecture of the data-driven graph generative model provides a powerful framework for generating graphs from temporal interaction data.
Improving Network Performance Through Data-Driven Graph Generative Models
Improving network performance by leveraging data-driven graph generative models offers several advantages. Firstly, it enhances network robustness and resilience, ensuring uninterrupted connectivity. Secondly, it optimizes network efficiency, allowing for faster data transmission and reduced latency. Moreover, it enhances the quality of service, providing a smooth user experience with improved reliability.
By leveraging these models, network administrators can better understand the underlying patterns and dynamics of temporal interaction networks. This knowledge can then be used to make informed decisions, adapt network configurations, and optimize resource allocation. Ultimately, the use of data-driven graph generative models empowers network management and facilitates the development of more efficient and reliable network infrastructures.
Conclusion
As we wrap up our discussion on “a data-driven graph generative model for temporal interaction networks,” it is evident that this innovative model holds tremendous potential in understanding and predicting complex temporal interactions. By leveraging the power of graph neural networks and incorporating data-driven techniques, this model enables us to grasp the underlying patterns and dynamics of temporal networks.
It offers a promising approach to analyze various real-world scenarios, such as social networks, transportation systems, and financial markets. With its ability to generate realistic temporal interaction sequences and effectively capture the evolving nature of networks, this model establishes a robust foundation for further advancements in network analysis and prediction.
By embracing data-driven approaches, researchers and practitioners can gain valuable insights into the interconnectedness and temporal dynamics of various systems. As we look ahead, it is important to continue exploring the potential applications and refining the methodologies of this model, ultimately leading to more accurate predictions and a deeper understanding of temporal interaction networks.
By embracing data-driven graph generative models, we pave the way for innovative solutions that have a lasting impact on various fields, opening up new avenues for research, analysis, and decision-making.